
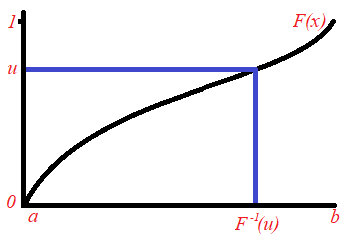
Gratiet LL, Cannamela C (2012) Kriging-based sequential design strategies using fast cross-validation techniques with extensions to multi-fidelity computer codes. Comput Methods Appl Mech Eng 349:360–377. Ĭheng K, Lu ZZ, Zhen Y (2019) Multi-level multi-fidelity sparse polynomial chaos expansion based on Gaussian process regression. Ĭhen S, Jiang Z, Yang S, Chen W (2017) Multimodel fusion based sequential optimization. Ĭhen S, Jiang Z, Yang S, Apley DW, Chen W (2016) Nonhierarchical multi-model fusion using spatial random processes. Ĭhen Z, Peng S, Li X, Qin H, Xiong H, Gao H, Li P (2015) An important boundary sampling method for reliability-based design optimization using kriging model.
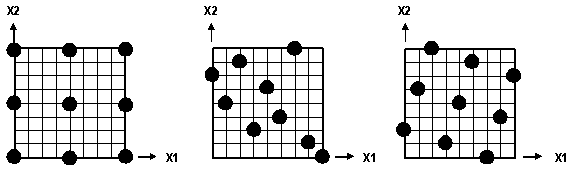
īiehler J, Gee MW, Wall WA (2015) Towards efficient uncertainty quantification in complex and large-scale biomechanical problems based on a Bayesian multi-fidelity scheme. The effectiveness and relative advantage of the proposed multi-fidelity sampling strategy in terms of efficiency is demonstrated by comparative studies using several numerical examples for uncertainty propagation and an airfoil robust optimization problem.Īhmar AM, Raisee M (2020) Multi-fidelity uncertainty quantification of film cooling flow under random operational and geometrical conditions. Furthermore, a sample density function is introduced to avoid the clustering of samples, which can prevent wastage of sample points and the singularity problem. With the proposed sampling strategy, the input location with the largest prediction error is identified as the new input sample point, and then, the multi-fidelity model with the largest CP index is selected for evaluation to reduce the computational cost as much as possible. To address this issue, a new maximum cost performance (MCP) sequential sampling strategy considering both the sample cost and accuracy improvement is proposed based on the recently developed multi-fidelity PC-Kriging (MF-PCK) approach. Systematically allocating sample points from multi-fidelity models to ensure both the accuracy and efficiency of the metamodel still remain challenging. However, previous studies mainly focused on multi-model fusion. To reduce the computational cost of uncertainty propagation, multi-fidelity polynomial chaos approaches have been developed by fusing a few expensive high-fidelity data points and many less expensive lower-fidelity data points to build a stochastic metamodel.
